ECVFSLHS_WA:
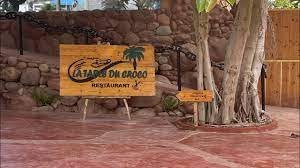
ECVFSLHS_WA is a term that may sound unfamiliar to most people. However, it is a topic that has been gaining increasing attention from researchers, particularly those in the field of machine learning and artificial intelligence. This article will provide a brief introduction to ECVFSLHS_WA and its potential implications for the future of technology.
What is ECVFSLHS_WA?
ECVFSLHS_WA is an acronym that stands for “Efficient Convolutional Neural Networks via Fisher Sparse Learning with Hard Shrinkage.” In simpler terms, it refers to a method of training machine learning models, particularly convolutional neural networks (CNNs), that aims to reduce the computational cost and memory requirements while maintaining high accuracy.
The idea behind ECVFSLHS_WA is to use a combination of techniques such as Fisher sparse learning and hard shrinkage to reduce the number of parameters in the model. This approach can result in faster and more efficient computations, which is particularly important when dealing with large datasets or complex models.
Why is ECVFSLHS_WA important?
The importance of ECVFSLHS_WA lies in its potential to make machine learning models more efficient and scalable. CNNs, for example, are commonly used for image and video processing, but they can be computationally expensive, especially when dealing with large datasets. ECVFSLHS_WA provides a way to reduce the computational cost and memory requirements without sacrificing accuracy, which can make these models more accessible and practical for a wider range of applications.
ECVFSLHS_WA can also have implications for the development of edge computing and the internet of things (IoT). These technologies rely on small, low-power devices that have limited computational resources. By making machine learning models more efficient, ECVFSLHS_WA can enable these devices to perform more sophisticated tasks, such as image recognition or speech processing, without requiring significant computing power.
Possible Applications of ECVFSLHS_WA
The potential applications of ECVFSLHS_WA are numerous and varied. Here are some examples:
Image and video processing: CNNs are commonly used for tasks such as object recognition, image segmentation, and video analysis. ECVFSLHS_WA can make these tasks more efficient, which can lead to faster processing times and better performance.
Speech processing: Speech recognition and natural language processing are other areas where CNNs are commonly used. ECVFSLHS_WA can help make these models more efficient and accurate, which can improve the overall performance of these applications.
Edge computing and IoT: As mentioned earlier, ECVFSLHS_WA can have implications for edge computing and IoT. By making machine learning models more efficient, these technologies can perform more sophisticated tasks without requiring significant computing power.
Conclusion
In conclusion, ECVFSLHS_WA is a promising approach to training machine learning models, particularly CNNs. By reducing the computational cost and memory requirements, it can make these models more efficient and scalable, which can have implications for a wide range of applications. As research in this area continues, we can expect to see more applications of ECVFSLHS_WA in the future.